As companies scramble to understand and deploy Generative AI, the much-hyped technology is proving to be a difficult beast to tame. That has created an opening for startups that can help enterprises refine their GenAI services to realize the potential for greater automation and efficiencies.
Adaptive ML, one of these new breeds of startups building an ecosystem of tools around Large Language Models, has raised a $20 million Seed Round to accelerate the development of a service that addresses this GenAI pain point. With co-headquarters in New York City and Paris, the startup must now turn an early beta into a solid product as the market for such services continues to evolve at a furious pace.
“When we first chatted with companies, we asked them what kind of difficulties they were having with their GenAI deployments,” said Adaptive ML Co-Founder and CEO Julien Launay. “Typically, we heard the same thing, which is that they tried something, but they are struggling to get the level of performance they want, and they don’t see a path forward to get to something that will deliver what they expect. What we said with our platform is that this is an environment that helps to get the results you want, to use the feedback to get the model to be better day after day, week after week.”

What is Adaptive ML?
Adaptive ML helps companies refine their GenAI models by analyzing user behavior and then automating the process of incorporating that feedback to adapt the model based on those interactions. The company’s Adaptive Engine targets enterprises that are deploying open-source LLMs across different business services. The goal is to improve the experience of these enterprises’ customers so the GenAI-powered services will be stickier.
Why?
While demand for LLMs has exploded, taking off-the-shelf models and adapting them to a specific use case is complex. The result is often features enterprises deploy that fall short of users’ expectations, driving little engagement.
OpenAI, which uses closed LLMs, has developed an intricate preference-tuning process that has helped its enterprise customers rapidly refine their models.
Optimizing an open-source LLM for a specific business application can be complex and expensive. Adaptive wants to make such tools more broadly available to enterprises using open-source LLMs such as Meta’s Llama or Mistral’s 7B.
Under The Hood
LLMs are trained using vast amounts of data and then refined by adding business-specific data. However, these more generic and off-the-shelf models don’t necessarily create production-ready models whose features are sufficiently aligned to the needs of businesses or their customers, according to Launay.
Adaptive Engine builds on this GenAI foundation through a continued system of fine-tuning and learning as people use the models. The platform leverages preference tuning which has typically been only accessible to researchers doing cutting-edge work because the tech can be hard to deploy correctly.
By creating an automated feedback loop, the Adaptive Engine helps align GenAI responses to users’ intent. Adaptive has achieved this through the development of a codebase called Adaptive Harmony that enables enterprises to deploy preference turning by adding just a few lines of code.
The Adaptive Engine also speeds this up by replacing the large number of humans who manually annotate data to drive the model’s learning capacity with an automated process known as Reinforcement Learning from AI Feedback (RLAIF). Once deployed, the Adaptive Engine automates A/B testing and then adapts the model based on those metrics.
“Very few people offer this method and not many people actually use and master it because it can be quite difficult,” he said. “We are packaging all of these so that any company can get a better value from their models.”
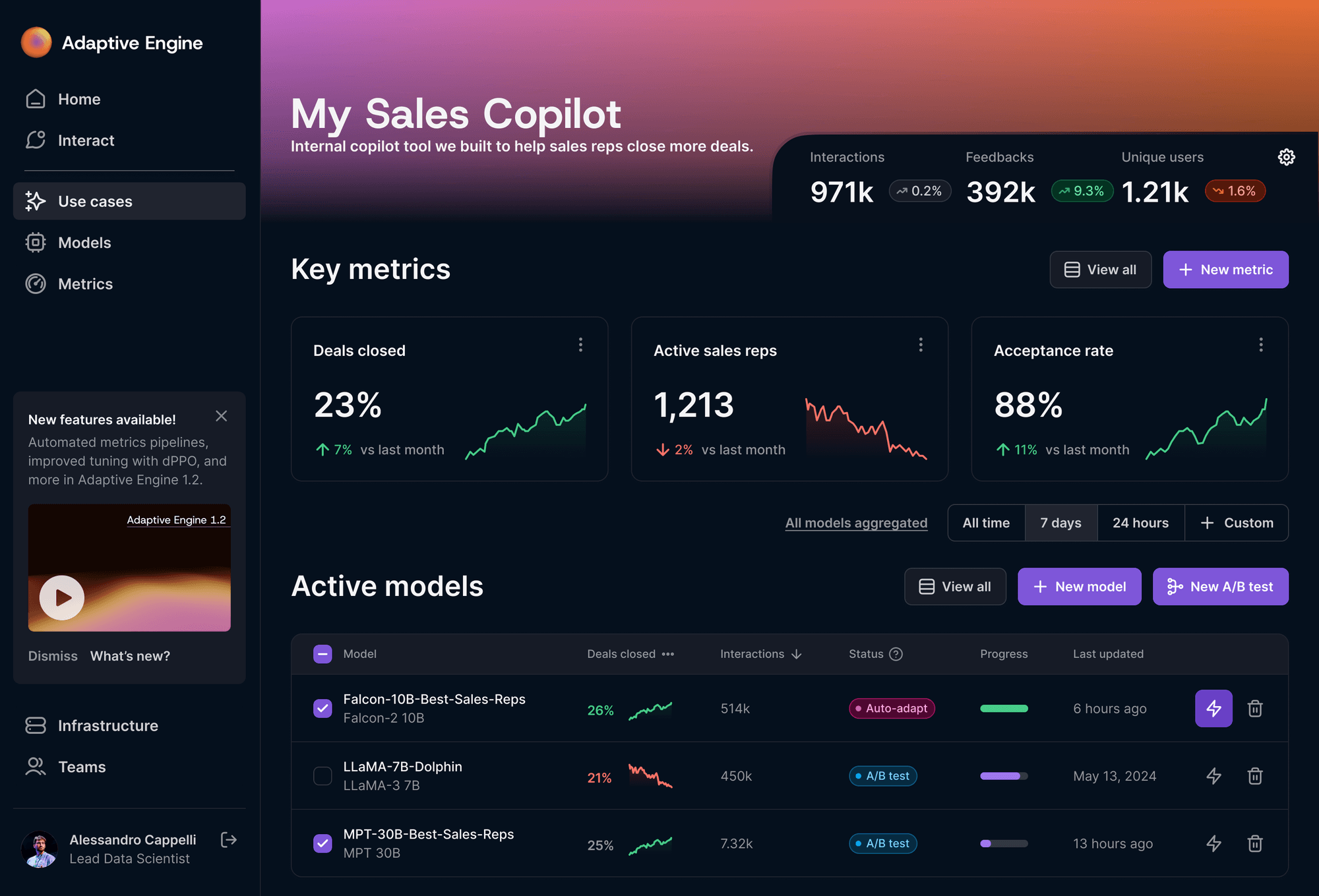
Along the way, the Adaptive Engine creates a set of metrics to help businesses optimize the tradeoff between the model size, the costs, and the improvements in services.
The company is also testing the idea of giving direct access to its Adaptive Harmony codebase so that others can experiment with preference tuning.
Who?
Adaptive has 6 co-founders, including 5 who have worked together for several years.
Launay and co-founders Daniel Hesslow (Research Scientist), Axel Marmet (RL Wizard), Baptiste Pannier (CTO), and Alessandro Cappelli (Research Scientist)all met while working at Paris-based GenAI startup LightOn. Later, they worked together on Falcon, an open-source LLM project at the Technology Innovation Institute in Abu Dhabi.
Last summer, several of them joined the Amsterdam office of Hugging Face, the AI model development platform started by several French co-founders in New York City. Hugging Face was in the process of adding Falcon to its platform.
The group decided to strike out on its own last fall and added former AWS engineer Olivier Cruchant (Lead Technical Product Manager) as the 6th co-founder. The group is in the process of relocating to NYC and Paris.
The Full Scoop...
Subscribe to get Adaptive ML's funding details, a breakdown of its investors, and its roadmap to raising a Series A round.